12. Why AI Transformation Efforts Fail - The Answers Might Not Surprise You (Part 1 of 2)

Brendan McLoughlin, President of e123, is participating in an executive education course at the Massachusetts Institute of Technology on Artificial Intelligence (AI) and its implications for business strategy. This is the twelfth in a series of blog posts where he shares the insights he is gaining and how they apply to health insurance distribution.
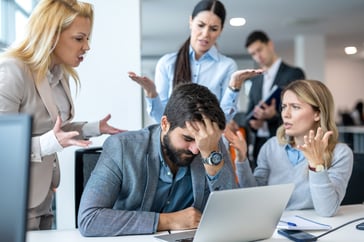
It might seem odd to refer to an Artificial Intelligence (AI) implementation as “transformation.” After all, in the context of health insurance distribution, AI is simply a set of technology tools deployed to achieve a business objective. However, like any major investment in technology, AI implementations should always have the ultimate goal of making an organization a better competitor. And having that level of impact on an organization involves far more than merely implementing new technology. Whether we call it reengineering, organizational transformation, business process improvement or innovation management, every successful AI implementation depends on some aspect of change management.
Harvard Business School professor John P. Kotter studied 100 companies in the midst of transformation and identified eight mistakes that business leaders make when trying to implement the change. In this issue, we will explore the first four, and discuss how they can apply to AI implementations in health insurance marketing organizations. Understanding and preventing these errors can dramatically improve the chances of success for any AI implementation and lead to lasting, meaningful organizational change.
Error 1 - Not Establishing a Great Enough Sense of Urgency
Effective AI implementations should always begin with aligning the initiative with a business objective, and successful change management begins with generating excitement and energy within the business focused on the “why” behind the change. Too often we see organizations have a natural inertia towards the status quo. Managers have spent years being rewarded for predictable results and minimizing risks. Implementing AI tools as additions or complete replacements for existing workflows represents significant change - one that managers and staff are likely to resist.
Great change in an organization often starts with leaders recognizing a threat or opportunity that requires change. The first step to make change happen is to communicate the urgent need behind the change. While this sounds relatively easy, Kotter shares that most companies fail at this very first step. By failing to create a sense of urgency within the organization, AI transformation initiatives are often doomed before they even start.
A great example I see in health insurance distribution surrounds commissions and their ability to attract, motivate and retain the best producing agents. Put simply, there is a battle in the marketplace right now for the highest producers. Health insurance executives commonly express that a key strategic focus is improving the quality of their distribution footprint by recruiting and empowering the best agents. And study after study suggests that timely, accurate, easy to understand commissions are a major tool in agent retention. Yet, I also commonly hear that most carriers and payers think their legacy commissions tools are “good enough”, that getting commissions mostly right is the status quo, and that their inefficient commissions systems are sufficient. In practice, line managers feel the cost and effort of becoming truly great at managing commissions is simply not worth the reward. This is clearly a case where the sense of urgency is just not there.
Error 2 - Not Creating a Powerful Enough Guiding Coalition
The most successful AI implementations in health insurance today support the idea of leveraging collective intelligence - the combined intelligence of humans and computers working together. It is no surprise then, that successful AI transformations rely on broad functional groups within the organization.
The challenge comes from the fact that organizational change often originates with a single leader or department rather than from a cross-functional coalition across the organization. The unfortunate natural inclination is for that single leader to “be the change agent!” For instance, a Fortune 500 company rolled out a major technology implementation by announcing that “IT is implementing a new enterprise system,” resulting in the project not being set up for success.
For a change initiative to be successful, the group of executives actively supporting it must be larger than the number of executives who are neutral or opposed to it. This can vary based on the size of the organization, but it can mean 10 to 50 senior leaders for example being on board and understanding their role in the initiative.
AI implementations that fail in due to error 2 are usually the result of not building a powerful, guiding coalition. Sometimes they expect change to be led by outside consultants or transformation departments. This is rarely successful without a powerful enough guiding coalition. The project can make apparent progress for a while, but sooner or later, the opposition gathers itself together and stops the change.
Error 3 - Lacking a Vision
Plans, directives, roadmaps and Gantt charts do not represent a vision. Self-described professional optimist Simon Sinek observed that “Dr. King gave the "I have a dream" speech, not the "I have a plan" speech. It's dreams that change the course of history.”
In every successful transformation effort that Kotter observed, the guiding coalition developed a picture of the future that is relatively easy to communicate and appeals to customers, stockholders, and employees. He suggests a rule of thumb - that leaders should be able to communicate the vision in under five minutes and generate interest and understanding. If you are driving an AI project and cannot communicate a vision this succinctly, you likely have more work to do.
Error 4 - Under-Communicating the Vision by a Factor of Ten
It takes effort to create a compelling vision that enlists the support of a majority of executives, let alone a majority of employees. It is simply not possible to force people to accept change against their will. In fact, employees would rather maintain the status quo - even if they are unhappy with the status quo - unless they truly believe positive change is possible.
It is vital that leadership reinforce the vision at every opportunity. WIth AI implementations, this goes behind hosting an all-hands meeting to share the vision. It means constant, open discussions of the project and its goals, including difficult conversations about how AI implementations can impact jobs. While leaders may naturally shy away from these tough topics, the research shows that communication comes in both words and deeds, with the latter often the most powerful.
I sometimes like to challenge my organization with a Big, Hairy, Audacious Goal (BHAG). When it comes to understanding a vision for change, Kotter suggests that 75%, or three out of every four employees should be able to understand and articulate the goal of any organizational transformation. A BHAG like this might seem like a lofty ideal, but striving for this level of alignment is crucial to drive change, and with AI projects it might be the most important factor that leads to success.
Conclusion
As we've explored in this first part, the success of AI transformation in health insurance hinges not only on the technical implementation but also on how well organizations manage change. The common pitfalls highlighted by Kotter—such as failing to establish urgency, not building a strong coalition, lacking a clear vision, and under-communicating that vision—are all too prevalent. By addressing these issues head-on, organizations can greatly increase their chances of achieving a successful AI transformation. In the next part of this two part series, we will dive into the remaining errors and discuss further strategies to ensure that your AI initiatives are not only implemented, but also embraced across the organization.
Want to learn more about e123 and the future of AI in insurance distribution? Get in touch here.
For prior posts in this series, click here or below: